Via Lactea Project 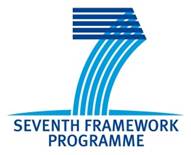
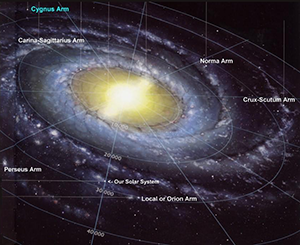
VIALACTEA will bring to a common forum the major new-generation surveys of the Galactic Plane from 1um to the radio, both in thermal continuum and in atomic and molecular lines, from Europe-funded space missions and ground-based facilities, to engage one of the fundamental challenges in Galactic astronomy: to quantify Galaxywide the relationship between the physical agents responsible for the onset and the regulation of star formation in a spiral galaxy and the resulting Rate and Efficiency of star formation, and obtain a "star formation recipe" that will be a cornerstone to trace the star formation history of galaxies back to their formation.
The new state-of-the-art Milky Way paradigm is offering today, for the first time, the possibility to deploy a coherent science analysis methodology that can be uniformly applied from the Galactic Center to the outskirts of the Galaxy. A homogeneous and inter-calibrated evolutionary classification of the cold and dense clumps hosting young forming clusters at a variety of evolutionary stages will allow to deliver a new 3D model of the Galaxy, mapping the essential critical parameters like column density thresholds, rate and efficiency of star formation in the Galaxy.
To make such an analysis possible in a timely and effective fashion, we will develop a suite of next-generation 3D-visualization tools that will integrate visual analytics, on-the-fly handling of multi-SED radiative transfer modelling and data mining/machine-learning technologies to incorporate the astronomer's know-how into a set of supervised workflows with decision making capabilities.
The focus on research and analysis of data obtained from European space missions in combination with data from Europe-funded ground facilities, will enable time-effective exploitation of steradiant-scale multi-wavelength Galactic Plane surveys through new 3D-based visual analytics frameworks, making VIALACTEA's objectives timely and totally relevant for FP7-SPACE-2013-1.

The DAME contribution
The building blocks of the scientific analysis methodology outlined in the ViaLactea project to yield basic results like Star Formation Rate and Efficiency, are currently carried out by the astronomer by directly evaluating with visual inspection each and every step of the process. Furthermore, the science foreseen to be produced in the proposed project is commonly understood to be data-intensive or data-driven. That is why data mining joined with robust machine learning based methods scalable and capable to capture the complexity of the data need to be designed and deployed.
Machine learning is able to classify the shape features into groups as well to derive and extrapolate astrophysical quantities and photometric relations for huge sets of multi-band and multi-epoch object catalogues, from a given base of knowledge (ground truth or known samples).
The integration and exploiting of data-mining and machine-learning technologies will enable developing new tools that incorporate data products and the astronomer's know-how into a set of supervised workflows with decision-making capabilities to carry out building of Spectral Energy Distributions, distance estimate and Evolutionary classification of hundreds-of-thousands of star forming objects on the Galactic Plane.
The contribution of our Team is structured under the form of dedicated data mining solutions to well-identified project tasks as well as the integration of all resources available in the project and possible/required connections among various WPs and tasks. It goes without saying that each of these tools would require to include different levels and times of contribution along the project evolution, in order to provide system integration solutions and interfaces to fulfil both scientific and infrastructure requirements within the project, according to its contemporary status of maturity. The evolution of these solutions is mainly related to the level of detail concerning both the scientific requirements and the specification of the proposed solutions, in particular, by our side, in terms of exploration of data mining techniques candidate to satisfy the requirements.
More specifically, the current contribution has been focused on the preliminary analysis, design and development of some important tasks, foreseen to be approached by data mining and machine learning methodologies:
- The compact source band-merged extraction
- Optimization of filamentary structure detection and Filaments spine extraction
- Evolutionary classification of SF sources
The INAF OACN Team is composed by:
- G. Riccio - Physics PhD
- S. Cavuoti - Physics PhD
- A. Mercurio - Astronomer
- M. Brescia - Astronomer